NMITE academics James Atuonwu and Parakram Pyakurel speculate on how the principles of science and engineering could be utilised to improve education delivery.
What if we told you that how we teach engineering could be engineered itself? That’s the idea behind the concept of Pedagogical Engineering (PE) being pioneered by experts at NMITE (New Model Institute for Technology and Engineering). We’re not just training engineers—we’re re-engineering how engineering is taught.
So, What Is Pedagogical Engineering?
At NMITE, we believe education isn’t just about transferring knowledge; it’s about crafting authentic learning experiences for a changing world. At its core, Pedagogical Engineering is envisioned as a transdisciplinary practice that sits at the intersection of three distinct domains:
- Process Science – understanding how things change [1]
- Systems Science – understanding how elements interact [2]
- Pedagogy – understanding how people learn [3]
Together, they offer a new way to deliver teaching and learning—where students are viewed as dynamic participants in a learning system, and teaching as the engineering of conditions that guide their transformation to work-ready, world-conscious professionals.
Process Science: Learning as Transformation
Think of it this way: just as raw materials are transformed into valuable products in an industrial process, students enter an educational system in one state and emerge in another—with new skills, perspectives, and capabilities.
In Process Science, we study how different variables affect that transformation. In terms of pedagogy, we ask:
- What teaching methods (similar to variables in process science) drive deeper learning?
- How do we “tune” the inputs (resources, teaching strategies, environment) for the best student outcomes?
This metaphor makes learning measurable, modellable, and optimisable.
Systems Science: Learning as Interaction
But education isn’t a conveyor belt—it’s a complex, interactive system. That’s where Systems Science comes in.
In any learning environment, countless agents and variables are at play:
👩🏽‍🏫 Teachers
👨‍🎓 Students
📚 Content
đź§ Cognitive modes and differences
đź’¬ Social interactions
đź’ˇ Institutional policies
These interact in nonlinear ways, creating feedback loops and emergent properties—outcomes that arise not from any one part of the system, but from how parts interact. By modelling these interactions, we can understand why some teaching strategies succeed in one context and fail in another—and how to design adaptive, inclusive, resilient learning environments.
Pedagogy: The Human Science
Of course, traditional pedagogy remains vital—it’s our bridge to the human experience of learning. But when combined with the precision of process science and the depth of systems thinking, pedagogy becomes not just art, but science.
Pedagogical Engineering lets us:
- Uncover underlying mechanisms and patterns of teaching and learning
- Design learning journeys with intent and precision
- Simulate and test educational models before deploying them
- Continuously improve curricula based on feedback and outcomes
Why Modelling?
At the heart of the underpinning themes of Pedagogical Engineering is modelling—not the catwalk kind, but systems modelling (mechanistic, data-driven, which could involve Artificial Intelligence (AI) integration [4], and hybrid). These models help us understand how different factors affect student learning. Examples include:
- How learning environments evolve over time
- How different teaching strategies affect outcomes
- What combinations of methods best support diverse learners
An AI integration Example
This integrated approach isn’t just conceptual—it’s our ongoing work. One recent project uses Natural Language Processing to analyse open-text student feedback and is already yielding insights informing the redesign of lesson plans, creating a direct pathway from student voice to curriculum improvement [5].
Think of it as turning feedback into a blueprint.
This is Pedagogical Engineering at work:
🔍 Data → 📊 Insight → 🛠️ Design → 🔄 Improvement
Instead of manually sifting through comments, we are currently building a machine model that could:
- Uncover themes (e.g. support, pace, practical application) through topic modelling
- Classify new feedback into these themes for trend analysis
- Visualize insights for educators and curriculum teams to act on
The end goal? A closed feedback loop system for automated lesson planning and curriculum optimisation, grounded in real student experience.
A Vision for the Future
This isn’t just a one-off project. It’s part of a broader research vision exploring a variety of areas such as:
- Agent-based models of student-teacher-content dynamics
- Fuzzy logic systems for more equitable assessment
- Digital twins of learning environments to test curriculum changes before deployment
- Energy-based metaphors to analyse student workload and engagement
We’re not trying to reduce education to codes and algorithms. We’re trying to design it with intention—so it works better, for more people, more of the time.
Why This Matters Now
In a world that’s changing faster than ever, engineering education and indeed, education in general, must keep up. Students demand relevance. Institutions demand results. Society demands adaptability. Pedagogical Engineering offers us a way of rising to these challenges—not with slogans, but with tools. This is more than reform. It's re-engineering—from first principles, with learners at the centre.
As this great quote from Sir Winston Churchill reminds us:
“To every man there comes in his lifetime that special moment when he is figuratively tapped on the shoulder and offered a chance to do a very special thing, unique to him and fitted to his talents…”
For us at NMITE, that moment is now!
References
[1] vom Brocke, J., van der Aalst, W.M.P, Grisold, T., Kremser, W., Mendling, J., Pentland, B., Recker, J., Roeglinger, M., Rosemann, M. Weber, B. (2021). Process Science: The Interdisciplinary Study of Continuous Change. Available at SSRN: https://ssrn.com/abstract=3916817 or http://dx.doi.org/10.2139/ssrn.3916817
[2] Hieromymi, A. (2013). Understanding Systems Science: A Visual and Integrative Approach. Systems Research and Behavioural Science 30 (5), 580-595.
[3] Lo, M.L., Marton, F., Pang, M.F. and Pong, W.Y., 2004. Toward a pedagogy of learning. In Classroom discourse and the space of learning (pp. 189-225). Routledge.
[4] Walter, Y. (2024). Embracing the future of Artificial Intelligence in the classroom: the relevance of AI literacy, prompt engineering, and critical thinking in modern education. International Journal of Educational Technology in Higher Education 21:15. https://doi.org/10.1186/s41239-024-00448-3
[5] Atuonwu, J.C. (2025). Leveraging AI to enhance student learning: from identifying satisfactory experiences to informing curriculum design. Under Review.
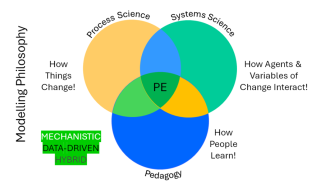